SalesRabbit • DataGrid AI
How can we leverage existing data to help sales teams identify qualified customers, cutting back on wasted canvassing time?
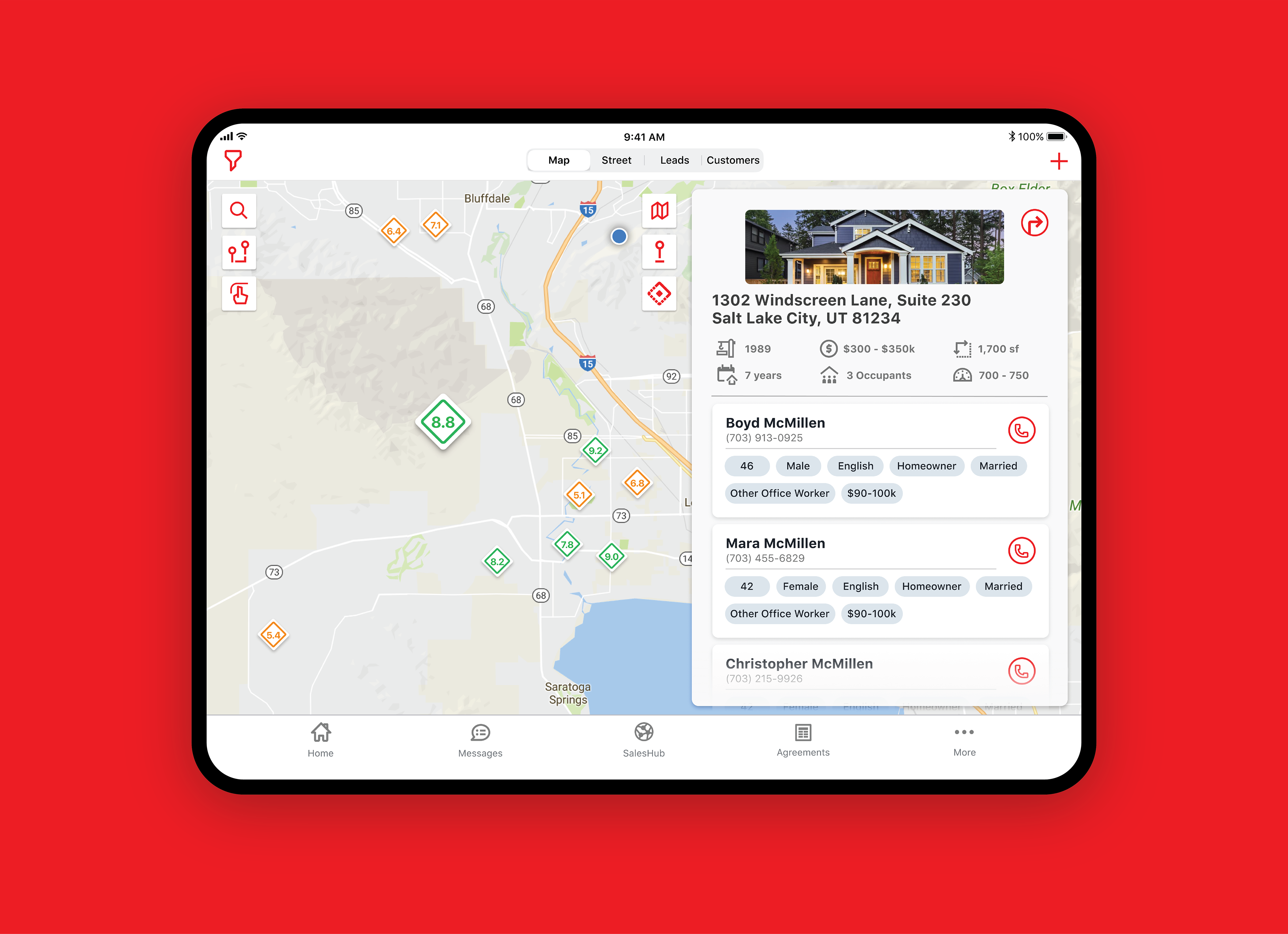
Summary
SalesRabbit is a sales enablement service and mobile CRM designed specifically for outside sales, its primary focus being lead management. By far the biggest time waster of lead generation for outside sales teams is cold canvassing prospects that aren’t ideal, or even qualified to move through a team’s sales funnel. While some businesses have come up with their own savvy solutions for targeting only qualified customers, most are left to unwelcomed cold canvassing.
Team Myself, CEO, 2-4 engineers (varied by project phase)
My Role Business strategy, UX research, UX design

Discovery
The idea behind DataGrid AI was born from an existing offering, DataGrid, so for this project I had the advantage of starting with knowledge of how the existing product was being used, and the feedback that customers had submitted. We would be partnering with a data provider for this product offering so thorough discovery was crucial, due to this I kicked off the project by interviewing multiple contacts from 8 companies of different industries and sizes, 4 being existing DataGrid customers, and 4 being prospects. These were some of the key takeaways:
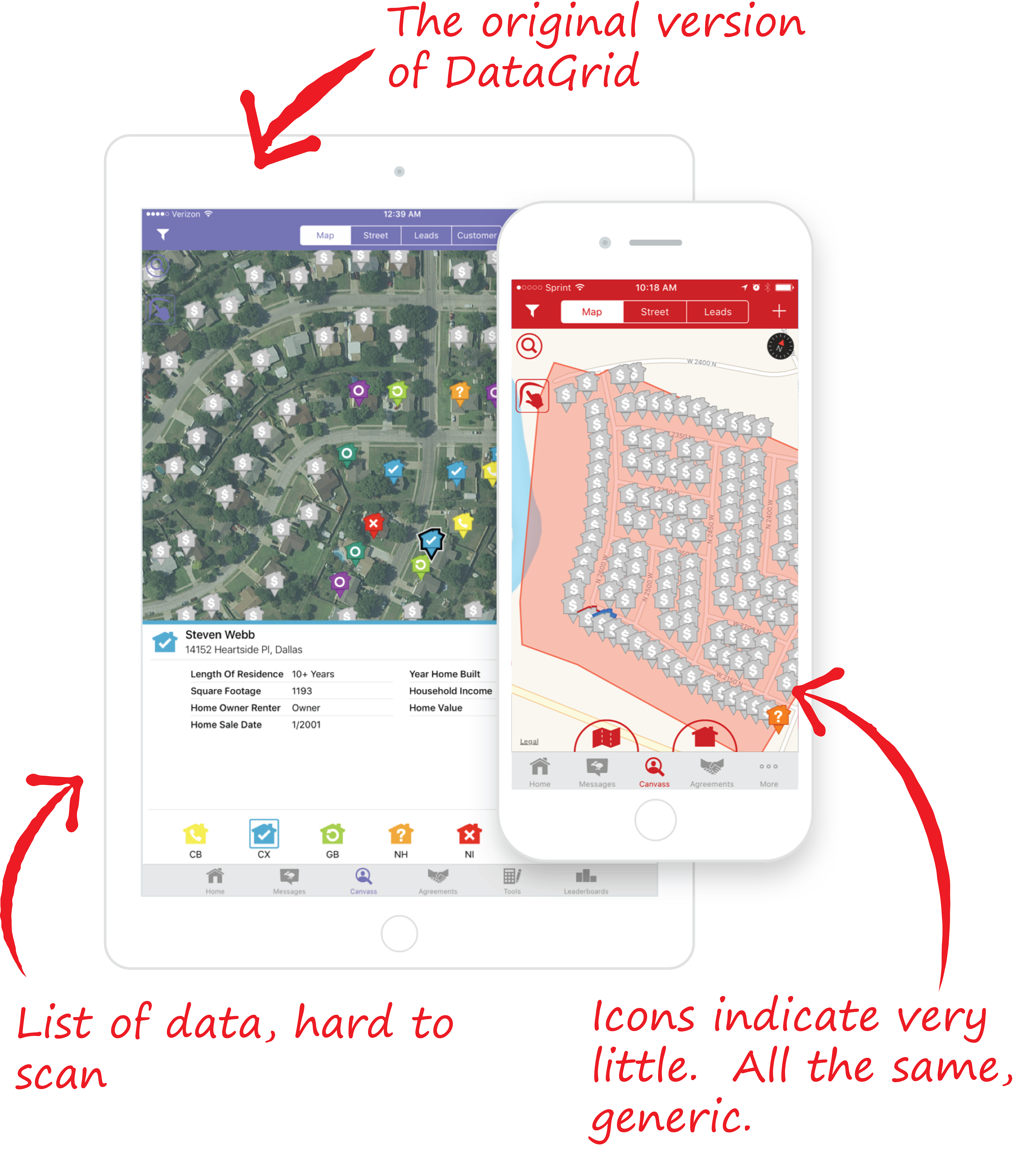
1•Success rates of canvassing are very low. The average conversion rates of sales canvassing was only about 1-2%. For a really good sales rep, they might hit 4%, but that difference generally came from spending much more time in the field.
2•Canvassing laws in Europe and Australia were tightening. One of the big pushes for DataGrid AI to move forward was because Europe and Australia were tightening laws on what days, and what times of day a sales rep could canvas. This was especially important for us to solve as one of our top 3 biggest contracts was with a B2B sales team based in Europe.
3•DataGrid in its current state was helpful, but hard to use. The existing product was not intuitive, so any new sales rep on the team had to be thoroughly trained before being able to utilize the tool.
Brainstorming
Initially I thought we would be limited by the data provided by our partner, Faraday, but what I found is that I actually had to spend a lot of time and effort vetting out which data points would be most essential across all of the markets that we served as they gave us access to over 4000+ buyer attributes. As a result I continued conversations with sales leaders, refining what would be presented in our MVP, while also being mindful of how to best structure the grouping of the data to optimize readability.
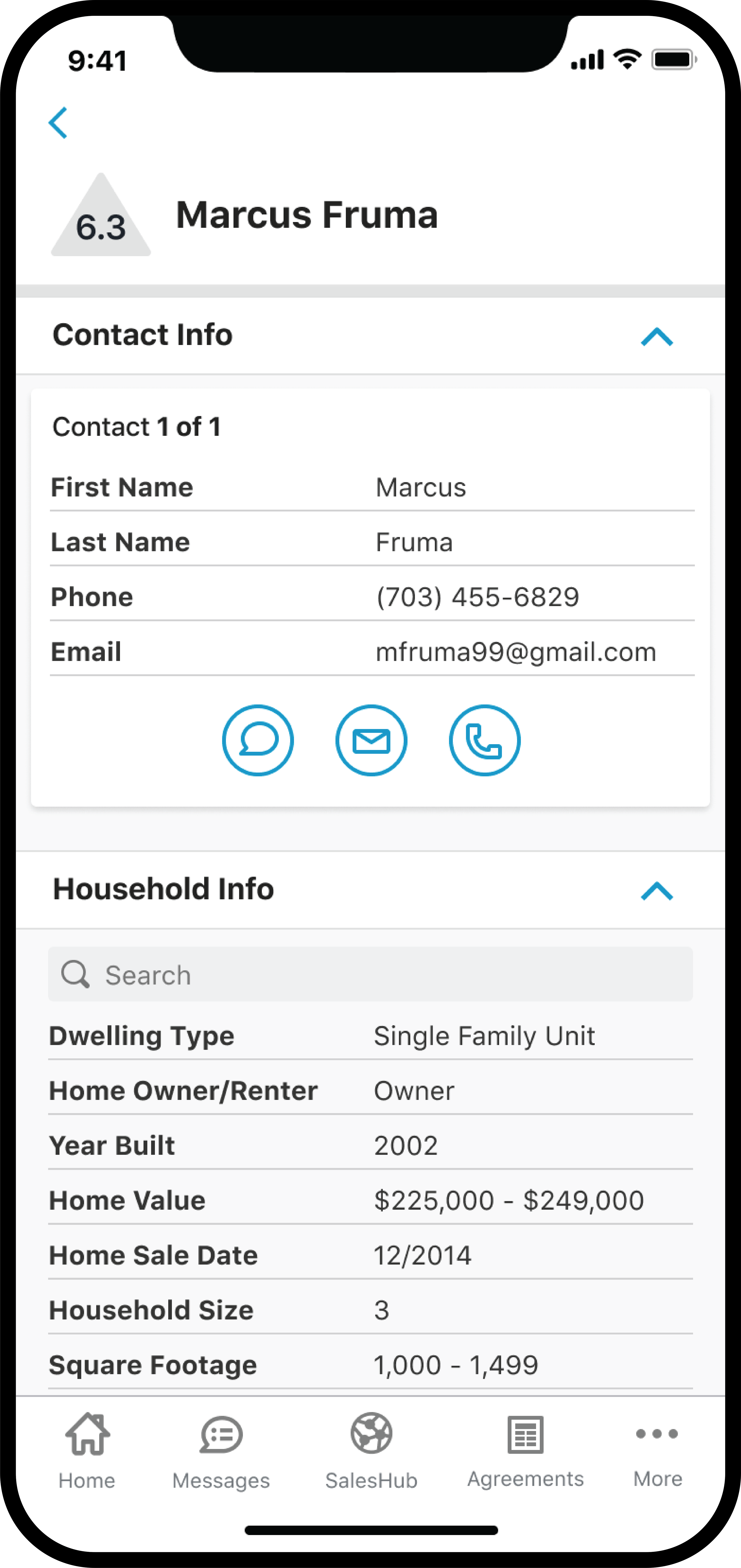
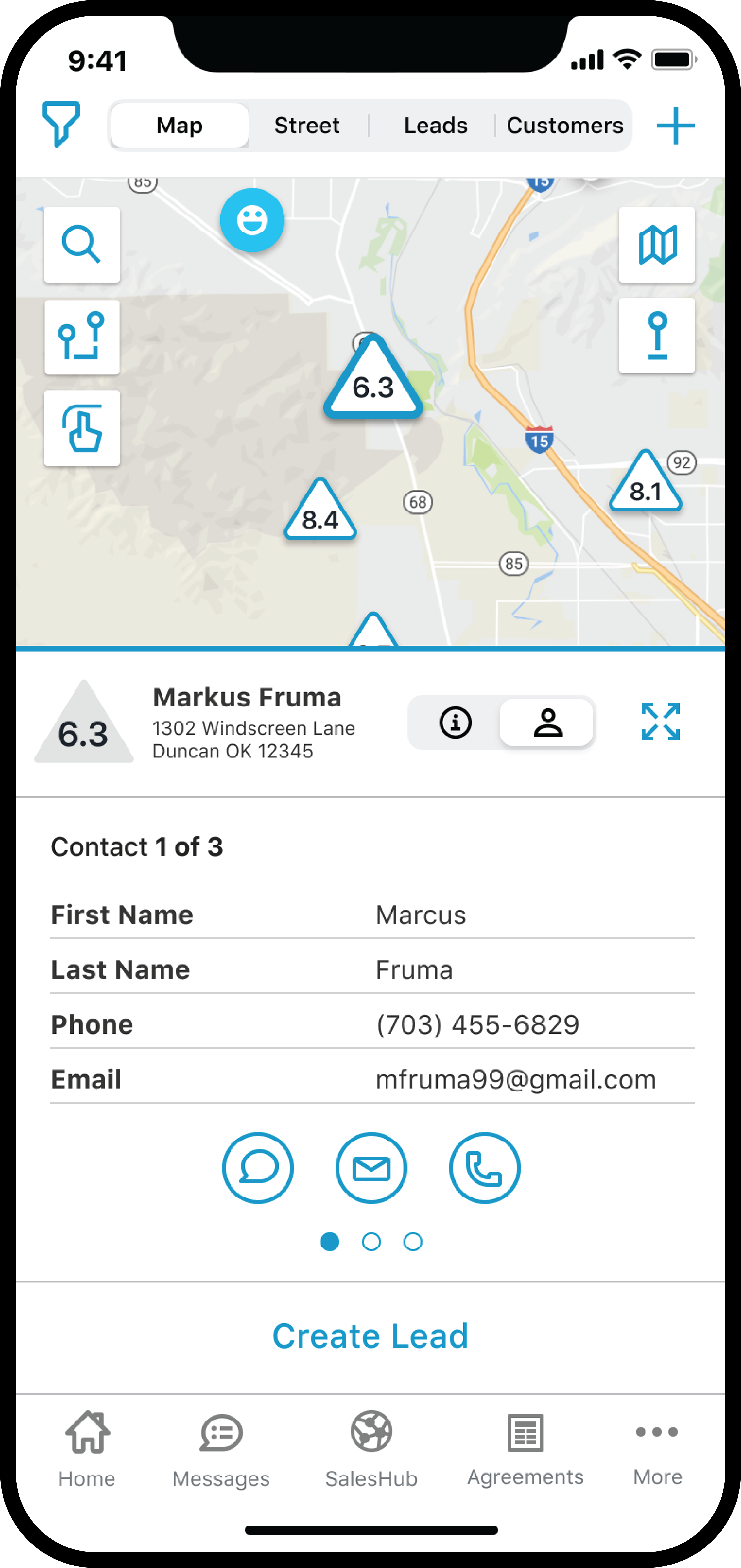
Filling in the Gaps
One of the most glaring drawbacks of the existing DataGrid offering was that each of the map objects looked exactly the same, causing reps to have to drill in to make the tool actionable. Additionally, once they did open up the details, they were just presented with a long list of data which was not easily scannable. In identifying this I set out to accomplish these main objectives:
1•Indicate the buyer propensity of the lead just from viewing the map.
2•Group the buyer data in a way that made it easily readable.
3•Make moving DataGrid AI prospects through the sales funnel simple.
Buyer propensity scores were actually much easier to accomplish than I originally anticipated by offering settings that could be adjusted based on industry and size within user admin settings.
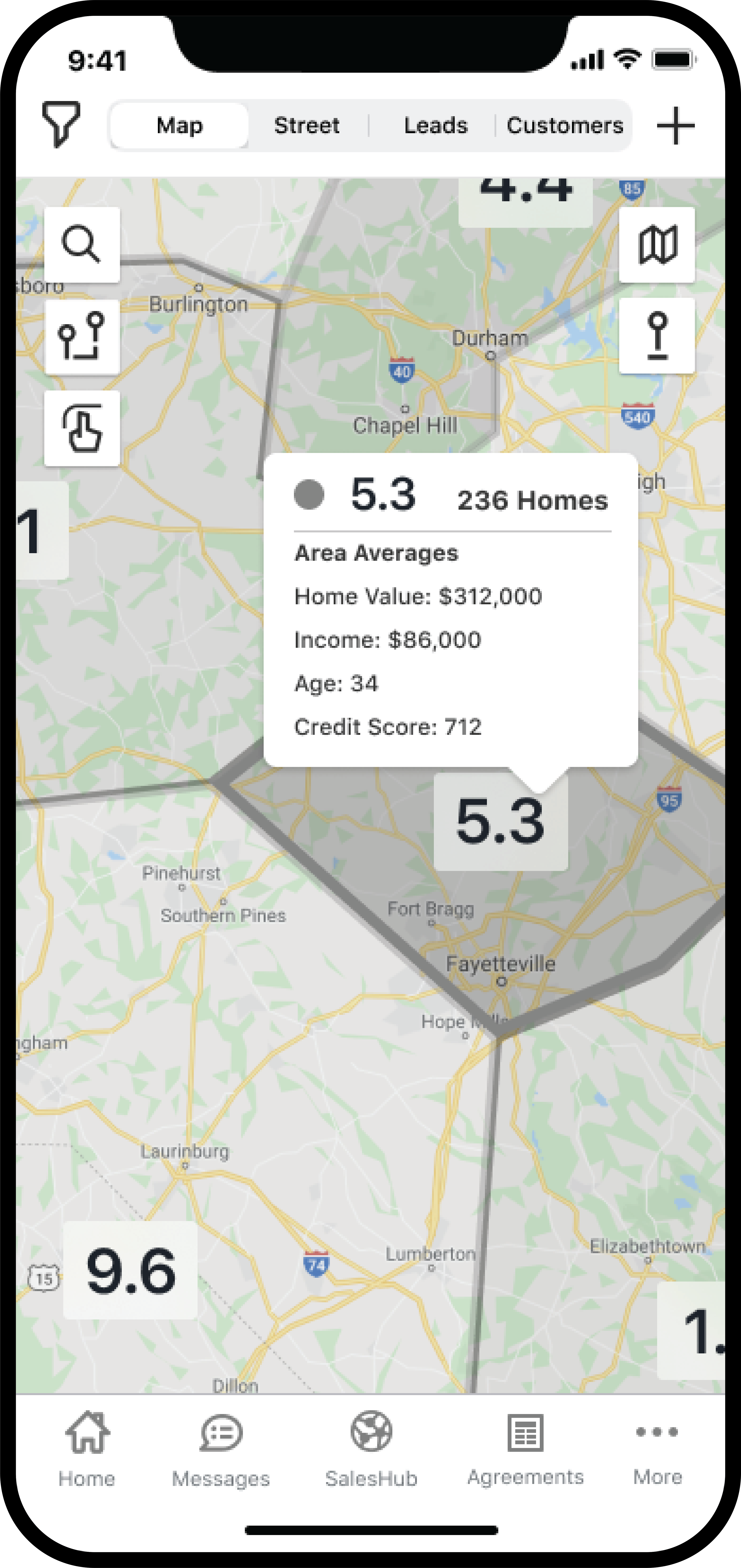
Iterative Innovation
Buyer propensity scores also sparked the idea of indicating area propensity scores on the map, accomplished by taking a combined score of prospects within county boundaries and creating custom .kmz files from that data. In doing so, our users not only had the ability to toggle a map overlay to indicate the most effective areas to canvas, but also the ability to assign those areas to specific sales reps within their organization.
Outcomes
After launch our team was eager to track usage of the new product, and we were blown away by the adoption. Within the first quarter after launch DataGrid AI had been leveraged to convert over 100,000 prospects to leads. We were also given qualitative feedback that DataGrid AI had significantly cut down on wasted canvassing time, though this proved hard to measure.
Not only that, but through continued communication with sales leaders we found that they had updated their methods of assigning reps to the field. Due to the fact that leaders could now visualize favorable areas they were able to be more efficient in where they assigned their top reps, what times of day they deployed them to the field, and increased the quality of training new sales reps as well.
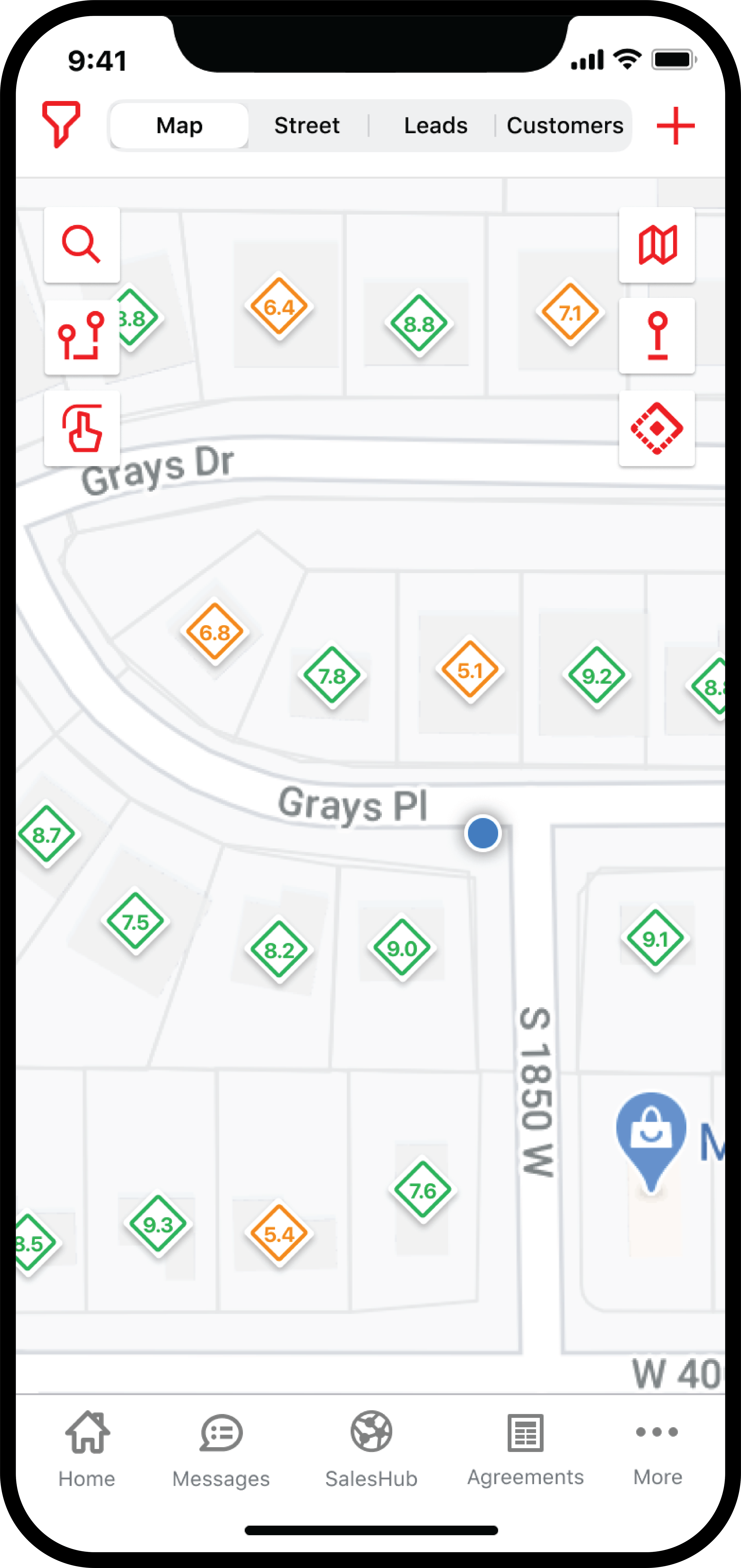
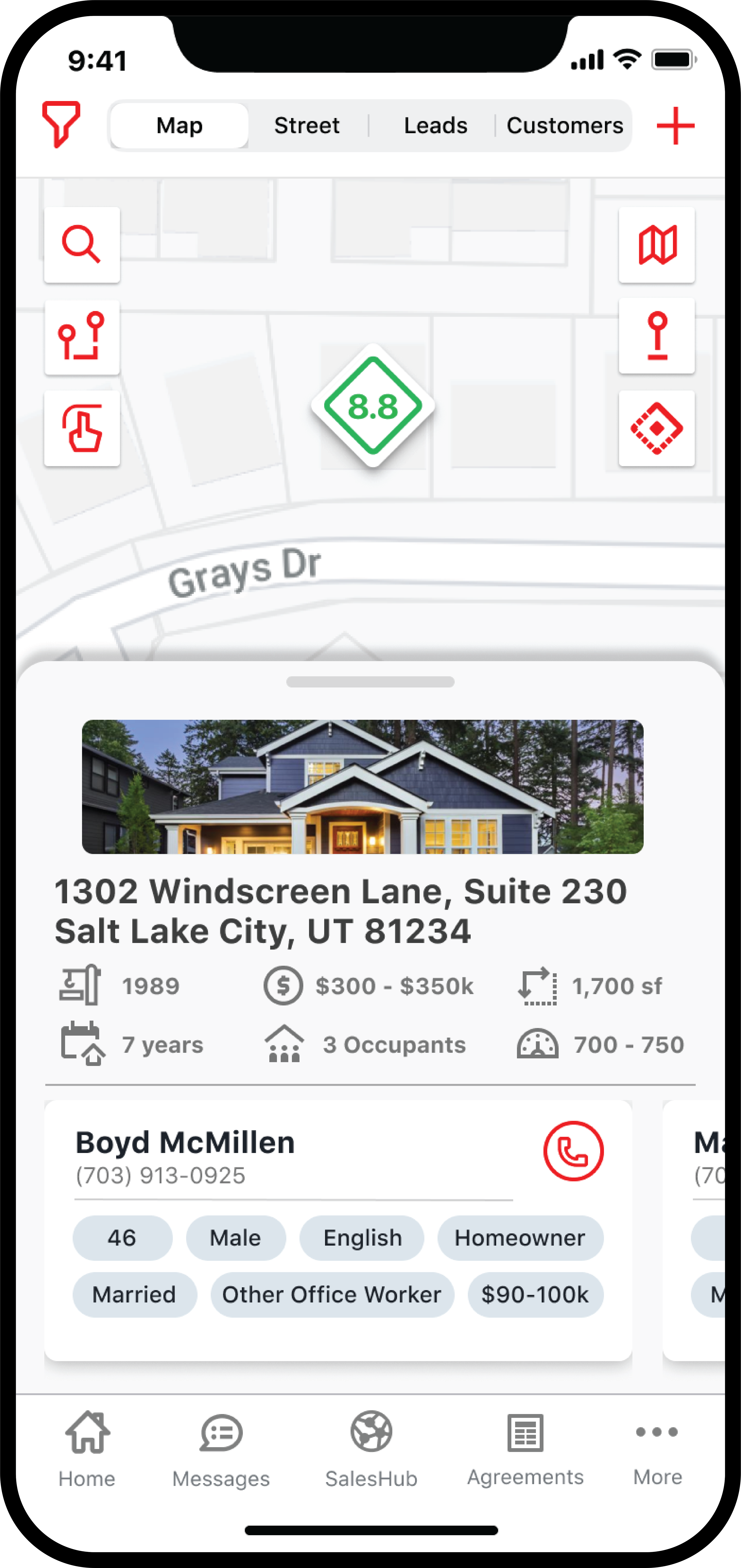
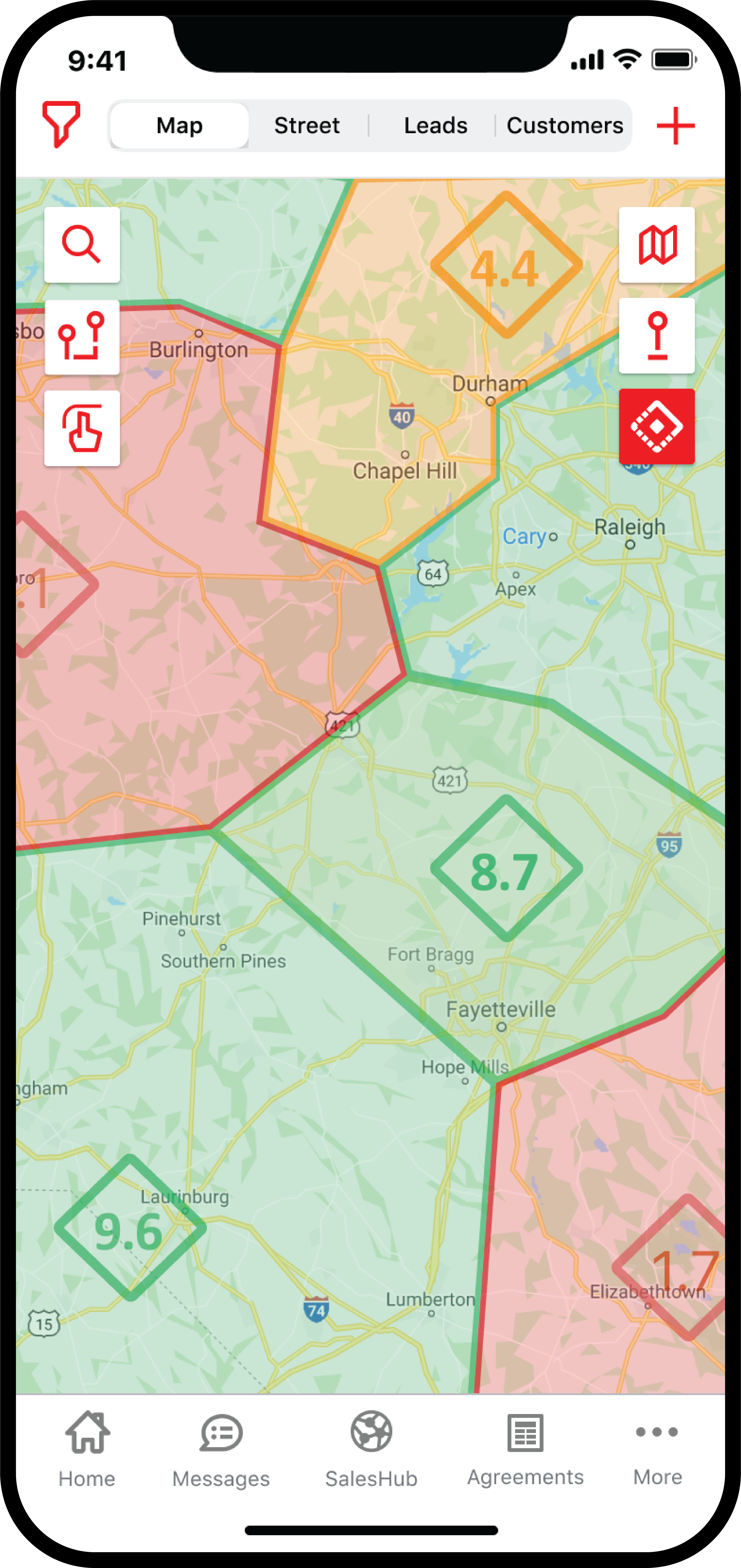
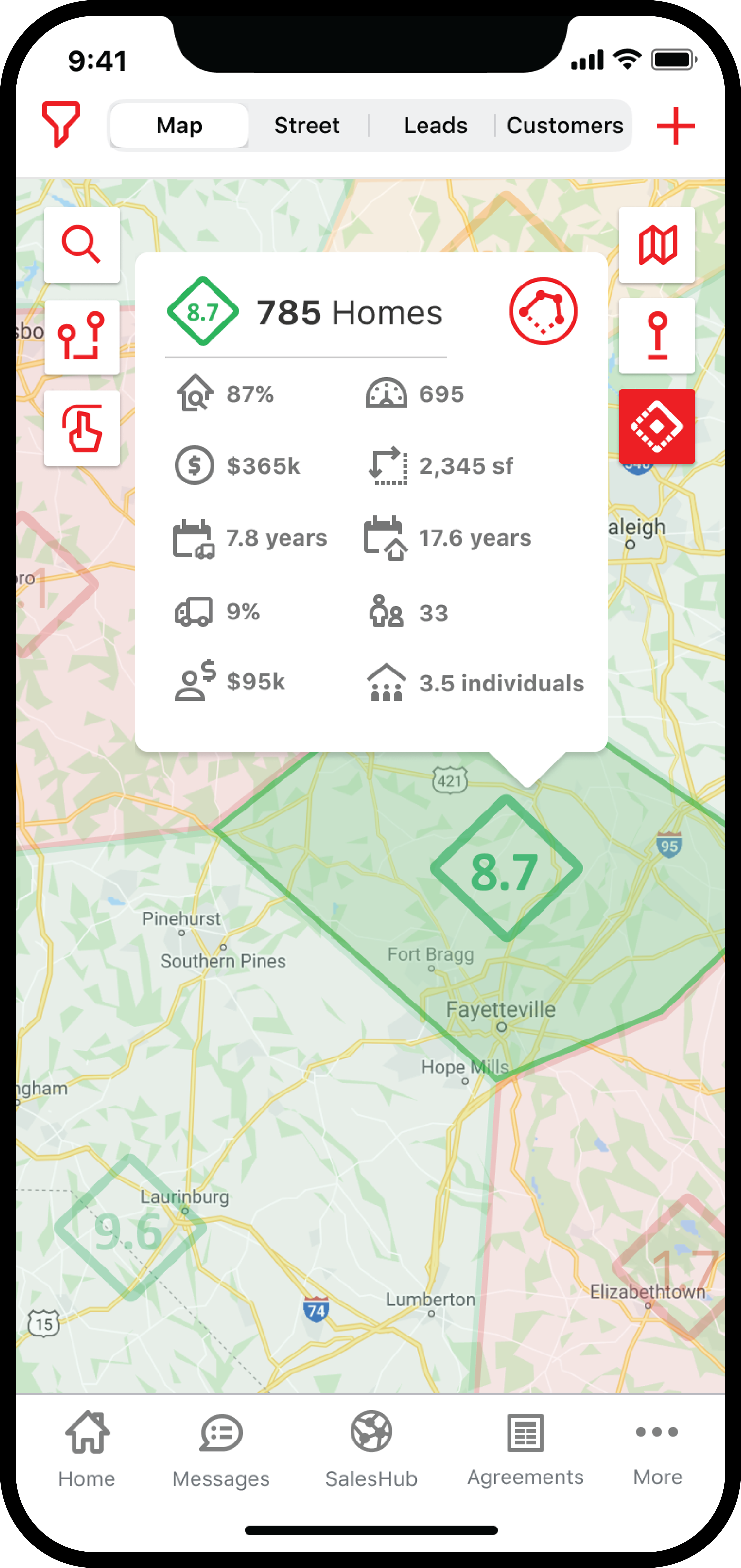